COVID-19: An example of why we need robust and fast process development
Updated: Apr 10, 2020
The current worldwide situation regarding the COVID-19 pandemic has shown all of us how vulnerable our daily life can be. In the case of such an unpredicted event, everyone must make trade-offs, personal and economic. That is why in such situations it is of the highest priority to react fast and develop a process to manufacture a vaccine as quickly as possible. However, prior to market entry, new drugs must be intensively tested in clinical trials, and even before the clinical trials the production process must first be developed and optimized.

Typically, during the process development step, design of experiments (DoE) is performed: critical process parameters (CPP) are defined and bioprocesses with different CPP setpoint combinations are performed, defining the so-called design space. Thereby, the necessary process understanding is generated and the optimal CPP settings for recombinant protein production in this design space are found. During this process characterization step, different process modeling strategies can be applied to extract valuable information in order to build a robust process set-up.
When looking exclusively at the development of a bioprocess, excluding effort for clinical trials, there are two major hurdles before a newly developed vaccine or any other drug is finally available on the market.
- Firstly, the development and optimization process required for different unit operations along the process chain is a very time and resource-intensive task. A lot of experiments have to be performed for each unit operation to find ideal conditions.
- Secondly, the developed process must be stable and robust enough to deal with variations in the input domain. The less time is invested in process development and screening, the less robust your process will be. Additionally, these experiments often have to be performed several times during upscaling.
“So, how can we get a robust process in a minimum amount of time?”
For the first task – enabling robust process development – we previously compared several modeling techniques for upstream process characterization. In contrast to downstream unit operations (like chromatography), where mechanistic descriptions are intensively used to accurately describe loading and elution conditions, the underlying mechanistic in an upstream process is very complex and difficult to describe. For this upstream showcase, we combined a minimum of process knowledge (equations) with a machine learning approach, called hybrid modeling, and compared the modeling results to the state of the art process endpoint descriptions, using DoE tools, and a purely data-driven approach.
For this evaluation, their performance, as well as model structure-related features, were evaluated. We demonstrated that hybrid modeling performed the best, in regards to the explanatory power and robustness, i.e., accuracy and preciseness.
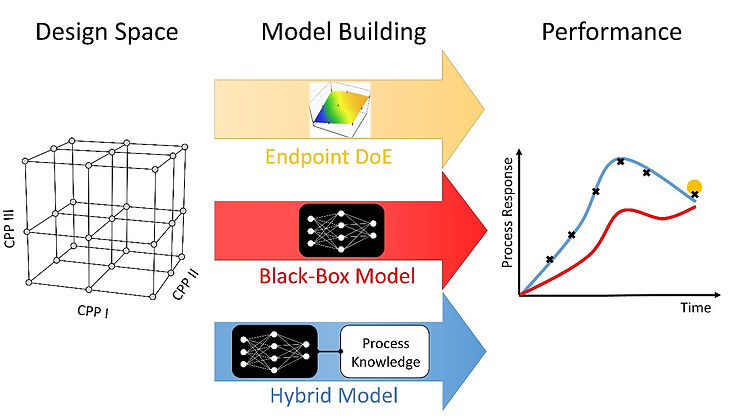
Even though we were able to highlight the advantages of using hybrid models, developed in our toolbox, for robust process development, the long development times still remain. As we showed that precise time-resolved modeling of an upstream bioprocess is possible, we considered using intensified design of experiments (iDoE) to speed up this characterization process. The concept of iDoE contains intra-experimental shifts, i.e., a switch from one of the earlier mentioned CPP setpoints in the design space to another one.
Below, an illustrated example of a design space with two CPPs, each investigated at three levels, is given.

By performing ordinary static bioprocesses, without changing any CPP setpoint, nine experiments are needed to characterize this design space and gather process understanding. However, learning from a design space can significantly be accelerated by performing intensified bioprocesses, applying two intra-experimental CPP shifts. Hereby, the same space is characterized by only a third of the experiments compared to the static approach. This leads to an accelerated process characterization by more than 66 %.
Of course, the given example is simplified. The setup of an iDoE requires many considerations, logical limitations, physiological constraints, and a well-thought workflow. Our latest manuscript will extensively cover this topic and is currently under review. We are eager to inform the scientific community soon regarding our findings, process knowledge and to contribute to speeding up bioprocess development for the development of new drugs and biosimilars.
We hope that we could give you an understanding of current challenges but also highlight our solutions to tackle these hurdles in process development,
stay safe!
Novasign team