Industry 4.0: Where are we, and how to accelerate the transition?
In order to move from industry 3.0 to 4.0 relevant process outputs (titer, product quality, certain impurities) have to be monitored and controlled in real-time. Process models provide the understanding of how input factors affect the outputs for every stage of the process in order to give recommendations of how to operate the process. Finally, model predictive control strategies must subsequently be applied in order to take action in such out-of-control occasions. Ideally, all unit operations are connected in a fully integrated way and the plant manages itself..
Although continuous bioprocessing (and the concept of pharma 4.0) is known for a long time, only a few companies like BiosanaPharma established an approved commercial process. Continuous bioprocessing offers a chance to increase efficiencies and simultaneously reduce costs significantly but it also has one major drawback: higher complexity. Advanced process understanding is necessary to operate a process chain of multiple operations for several weeks or months especially when accounting and reacting to unexpected process changes.
This understanding and the resulting decisions can only be covered by outstanding and most likely not available expert knowledge and/or process modeling. To recover a process from an in-process deviation back into the desired margins, one must know which screws to turn. Due to the huge complexity and potentially high number of possible interconnections, process modeling and advanced process control strategies like model-based or predictive control are the solution of choice.
How can such a control strategy be implemented and where to start? The unpleasant answer: early in the process development!
Process understanding is gathered in the early-stage development
While the manufacturing site is responsible to create the return of investment for any drug, the basis for a successful process most likely is created in the development department, aiming to set up a stable process, maximizing yield and product quality. During early-stage process development, the costs per experiment are comparably low. The introduction of significant process variability by changing the process inputs not only enables valuable process optimization but also sets the basis for models that can be used for scale-up and control purposes in the later stages. When thinking about process development and manufacturing in the concept of industry 4.0 we would need these algorithm-based control strategies. But how far are we?
When looking into development departments one of the most crucial steps for the creation of powerful process models is the request for central data storage and seamless integration of those data into modeling tools. This implies storing relevant process information (runtime information, metadata, and offline analysis) in a single platform. This platform is then used to fetch data for different modeling tools. Such a concept in combination with powerful modeling tools allows for easy integration of digital process twins because data is available in real-time and models can be continuously updated. Additionally, such a concept would allow biopharmaceutical companies to quickly develop self-learning powerful process models during the development without the need for data engineers and scientists to spend endless time searching and adapting all of the different data (from different sources). Although the concept of self-controlling plants is nothing new and has already been applied in various other industries, the concept is not applied in biopharma yet. Although we see a lot of effort in manufacturing to develop process models based on setpoint processes, the true value for the industry will only be enabled if the knowledge from the development department is conserved and successfully transferred by the use of such models. Here, a process design space is considered after risk analysis of relevant process inputs and outputs during early development. By performing experiments in a clever concept such as intensified DoE a lot of process knowledge is gathered with as few experiments as possible. During scale-up, the model with the conserved knowledge is redefined and ultimately used for monitoring and control.
Qubicon and Novasign to join forces
In order to approach industry 4.0, Qubicon and Novasign decided to join forces to enable faster implementation of relevant concepts. Qubicon sets the basis for data management and contextualization of all process-relevant information from R&D to GMP compliant production. Thereby, all online data is streamed automatically to the software and aligned with meta-information as well as offline analysis in the concept of ALCOA+. Based on this data, Novasign builds reliable hybrid models. Seamless export from all relevant data on a click is already available. This allows for model creation without the detour and error-prone task of setting different excel sheets together, containing all the relevant information, and significantly decreasing overall modeling effort. Once a model is created, new experiments can automatically be integrated into the already existing model structure, refining the model per experiment. This helps process engineers to learn much faster from their processes and conserve valuable knowledge in a hybrid model. During scale-up and manufacturing, the model is further refined and along the whole process/product lifecycle it can be used for process monitoring and automated process control. Therefore, the relevant model parameters are fetched in real-time and the model delivers responses about relevant process outputs (titer, product quality, impurities,…) for monitoring and control purposes within the Qubicon software.
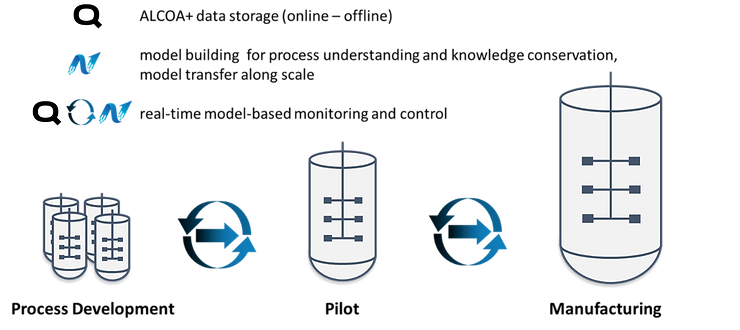
Figure 1. A schematic sketch of the tasks of Qubicon and Novasign for an upstream process from early-stage development until manufacturing and their connectivity.
Already in an advanced industry 3.0 approach, operators can then be notified or take action if a certain output threshold is reached (for example a certain biomass concentration that triggers induction or transfection of cells) or the process can automatically control itself by applying model-based or model predictive control strategies, enabling industry 4.0. Further, the output from one unit operation becomes the input to the next unit operation. If for all unit operations validated process models are established, the presented combined approach paves the way for automatic processes in an end-to-end manner.
Contact us or Qubicon if you are interested to learn more about the integration and applications.